SAS中Proc logisitc过程提供了很完善的logistic回归的分析功能,学习R中完成此过程只是想比较一下两个软件在完成此过程的差别。虽然有很多帖子介绍如何采用R完成logistic回归过程,但是都相对过于简单,对于以下常用细节很少涉及。
1、模型筛选方法
2、如何简单设定哑变量
3、针对分类变量,如何选取特定水平作为参考水平
4、如何简单输出OR值及置信区间
5、如何构建条件logistic回归过程
6、不同模型的预测效果比较
虽然之前有很多帖子比较SAS与R的差别,但是多是基于宏观层面的。通过比较实现某一具体过程的细微差别,估计更能体会两者的功能差异。
以下是自己学习的笔记,附有一些简单说明,供参考,希望对大家有帮助
1. library(stats)
2. help(infert) # Description of data
3. infert <- data.frame(infert)
4. str(infert) # Check type of variables
5. summary(infert) # Statistical summary
6.
7. ## Model1 Develop a simple logistic regression:
1. model1 <- glm(case ~ spontaneous+induced, data = infert, family = binomial())
2.
3. ## Model output
4. summary(model1) # Output summary information
5. confint(model1) # Output 95% CI for the coefficients
6. exp(coef(model1)) # Output OR (exponentiated coefficients)
7. exp(confint(model1)) # 95% CI for exponentiated coefficients
8. predict(model1, type="risk") # predicted values
9. residuals(model1, type="deviance") # residuals
10.
11. ## Model2 Develop a logistic regression adjusted for other potential confounders:
1. summary(model1) # Output summary information
2. confint(model1) # Output 95% CI for the coefficients
3. exp(coef(model1)) # Output OR (exponentiated coefficients)
4. exp(confint(model1)) # 95% CI for exponentiated coefficients
5. predict(model1, type="risk") # predicted values
6. residuals(model1, type="deviance") # residuals
7.
8. ## Model2 Develop a logistic regression adjusted for other potential confounders:
9. summary(model4)
10.
11. ## Model5 Conditional logistic regression
12. ## Conduct a subgroup analysis
13. ## "subset" is not aviable for "clogit"
14. ## create the subset first
15.
16. str(infert$education)
17.
18. infert1 <- subset(infert,education =="12+ yrs")
19.
20. model5 <- clogit(case ~ factor(spontaneous)+ factor(induced)+ strata(stratum),
21. data = infert1)
22. summary(model5)
23.
24. ## Model6 General logistic regression

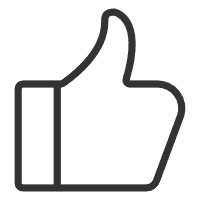
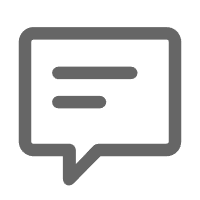
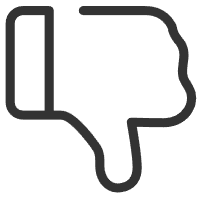
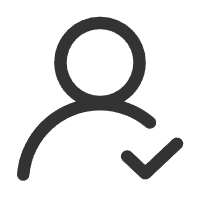
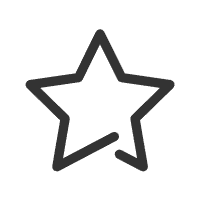
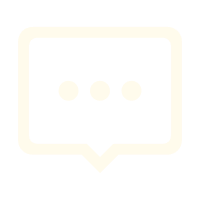

暂无数据