y是衔接需求的,y往往是1.预测误差 2.成本高 3.抽象 4. 业务危机 5. 业务增益
相关分析
plt.scatter(x='运动时间',y='体重',s=100,data=data,alpha=0.3)
sns.heatmap(data.corr(method='spearman'),cmap='GnBu_r')
sns.pairplot(data=data1.iloc[:,[0,1,2,3,4,5]],vars=['运动时间','骑行时间','体重'],hue='亲缘')
散点图主要看三点:1.相关系数 r 2. 斜率(回归) 3.异常值
相关系数在0.1以内几乎可认为不相关
相关系数在0.1~0.35之间为低相关
相关系数在0.35~0.5之间为中等弱相关
相关系数在0.5~0.7之间为中等强相关
相关系数在0.7~0.9之间为高相关
相关系数在0.9~1之间为高危相关
相关系数公式 y=βx ,β为相关系数的标准化后取值
线性回归分析
小数据
import statsmodels.formula.api as smf
result=smf.ols('体重~饮食+性别+亲缘+运动时间+骑行时间',data=data1).fit()
result.summary()#汇总结果
预测
x_new=pd.DataFrame([{'饮食':'加餐','性别':'男','亲缘':'很高','运动时间':3.22,'骑行时间':1.36}])
result.predict(x_new) #本模型预测必须在x的合理区间内,超过这个区间预测不准确
ols(最小二乘法)、正则化\梯度下降法算法对比
from sklearn.linear_model import LinearRegression,Lasso,SGDRegressor
x,y=data.iloc[:,1:],data.iloc[:,0]
reg=LinearRegression().fit(x,y)
print('OLS法:准确度%s和系数%s' %(reg.score(x,y),reg.coef_))
lasso=Lasso(alpha=0.2,max_iter=100).fit(x,y)
print('正则化法:准确度%s和系数%s' %(lasso.score(x,y),lasso.coef_))
sgd_reg=SGDRegressor(max_iter=100).fit(x,y)
print('梯度下降法:准确度%s和系数%s' %(sgd_reg.score(x,y),sgd_reg.coef_))
画框宽高比保证在1.25~1.6之间,plt.figure(figsize=(16,12))
plt.subplot(221)#2行2列的第1位置,位置按从左到右,从上到下的顺序数。
zres=(result.resid-result.resid.mean())/result.resid.std()
plt.scatter(result.predict(),zres)
plt.xlabel("predict")
plt.ylabel("zresid")
plt.axhline([0],color = 'r',ls="--")
plt.subplot(223)
x1=data.iloc[:,4]
plt.scatter(x1,zres)
plt.xlabel("x1")
plt.ylabel("zresid")
plt.axhline([0],color = 'r',ls="--")
plt.subplot(224)
x2=data.iloc[:,5]
plt.scatter(x2,zres)
plt.xlabel("x2")
plt.ylabel("zresid")
plt.axhline([0],color = 'r',ls="--")
误差和自变量x,因变量y 比较好的散点图的特点:
(1)随着ξ=0上下随机摇摆
(2)上下波动幅度几乎一致
(3)理论上呈圆形
数据波动不一致叫异方差,大方差/小方差<3是正常的,超出3表示有异方差
异方差检验
import statsmodels.stats.api as sms
res=sms.diagnostic.het_white(result.resid,exog=result.model.exog) #diagnostic 方法名为诊断,exog代表外生,方程式左侧y为内生,右侧为外生
print('LM:',res[0])
print('LM_p',res[1])
消除异方差
reslog=sms.ols('np.log(体重)~运动时间+骑行时间',data=data).fit()
reslog.summary()
sns.jointplot(reglog.resid,reslog.predict(),kind='reg') #reg|resid|kde|hex

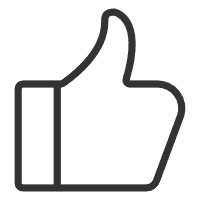
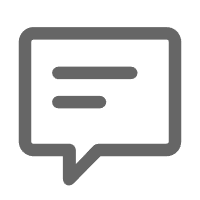
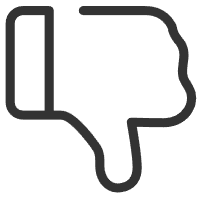
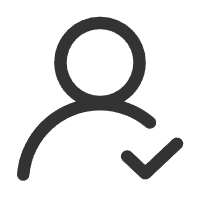
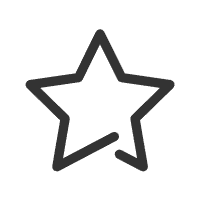
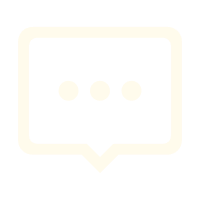

暂无数据