2020-06-12
阅读量:
2049
SVD-矩阵奇异值分解 —— 原理与几何意义
1.简介
SVD 全称:Singular Value Decomposition。SVD 是一种提取信息的强大工具,它提供了一种非常便捷的矩阵分解方式,能够发现数据中十分有意思的潜在模式。
主要应用领域包括:
- 隐性语义分析 (Latent Semantic Analysis, LSA) 或隐性语义索引 (Latent Semantic Indexing, LSI);
- 推荐系统 (Recommender system),可以说是最有价值的应用点;
- 矩阵形式数据(主要是图像数据)的压缩。
2.线性变换

3. SVD 推导



.4 实现代码
以下是基于 python 的 numpy 库实现的 SVD 矩阵分解的代码,用于实现图像压缩的功能。
代码链接 : https://github.com/zlxy9892/ml_
# -*- coding: utf-8 -*-
import numpy as np
import numpy.linalg as la
import matplotlib.pyplot as plt
from sklearn import datasets
from skimage import io
def getImgAsMat(index):
ds = datasets.fetch_olivetti_faces()
return np.mat(ds.images[index])
def getImgAsMatFromFile(filename):
img = io.imread(filename, as_grey=True)
return np.mat(img)
def plotImg(imgMat):
plt.imshow(imgMat, cmap=plt.cm.gray)
plt.show()
def recoverBySVD(imgMat, k):
# singular value decomposition
U, s, V = la.svd(imgMat)
# choose top k important singular values (or eigens)
Uk = U[:, 0:k]
Sk = np.diag(s[0:k])
Vk = V[0:k, :]
# recover the image
imgMat_new = Uk * Sk * Vk
return imgMat_new
# -------------------- main --------------------- #
A = getImgAsMatFromFile('D:/pic.jpg')
plotImg(A)
A_new = recoverBySVD(A, 30)
plotImg(A_new)
这里用小黄人的大头照做个例子看看:
原图:

设置 k = 10,得到的压缩图:

k = 20:

k = 30:

继续增加 k 值,将会得到越来越接近原始图的压缩图。
5 参考资料

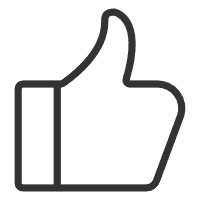
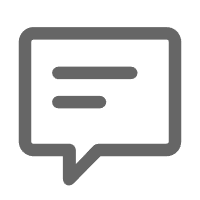
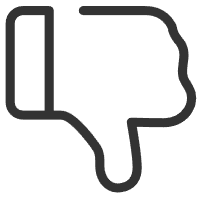
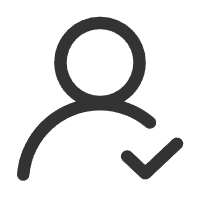
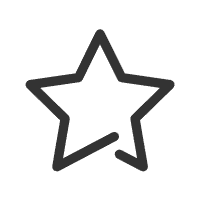
评论(0)
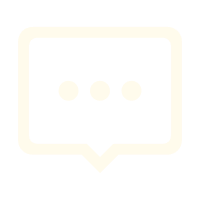

暂无数据
推荐帖子
0条评论
0条评论
0条评论