2021-04-26
阅读量:
1716
为什么需要交叉验证这种方式
当仅有有限数量的数据时,为了对模型性能进行无偏估计,我们可以使用k折交叉验证(k-fold cross-validation)。 使用这种方法时,数据被分成k份数目相等的子集。我们构建k次模型,每次留一个子集做测试集,其他用作训练集。如果k等于样本大小,这也被称之为留一验证(leave-one-out)。
在kaggle上,一些数据集是已经对训练集和测试集进行了区分,这种情况我觉得是不用再进行数据集的切分,直接进行交叉验证的,只需要直接在测试集上进行测试即可。
需要交叉验证的场景,是指那种数据量比较少的情况下,仅仅有训练数据,训练误差并不能体现出你相应的性能,要充分利用有限的数据。
进行多次验证或者将测试集的比例增大,可以在统计学上提高算法的置信度。

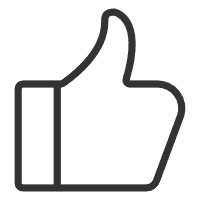
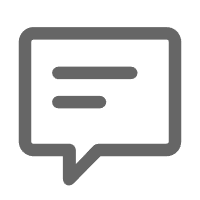
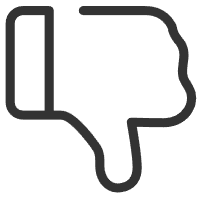
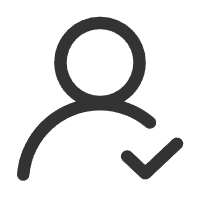
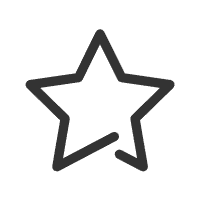
评论(0)
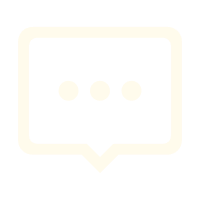

暂无数据
推荐帖子
0条评论
0条评论
0条评论